投資
4投資退出
45基金
1合作夥伴和客戶
10關於麻省理工學院媒體實驗室
麻省理工學院媒體實驗室超越了已知的界限和學科,鼓勵看似不同的研究領域進行最非傳統的混合和匹配。它創造了發生在邊緣的顛覆性技術,開創了諸如可穿戴計算、有形界麵和情感計算等領域。今天,實驗室的教職員工、研究人員和學生在24個研究小組中開展了350多個項目,從治療神經疾病的數字方法,到可持續城市的可堆疊電動汽車,再到可以“看到拐角”的先進成像技術。實驗室致力於超越顯而易見的問題,提出尚未提出的問題——這些問題的答案可以從根本上改善人們的生活、學習、表達、工作和娛樂方式。
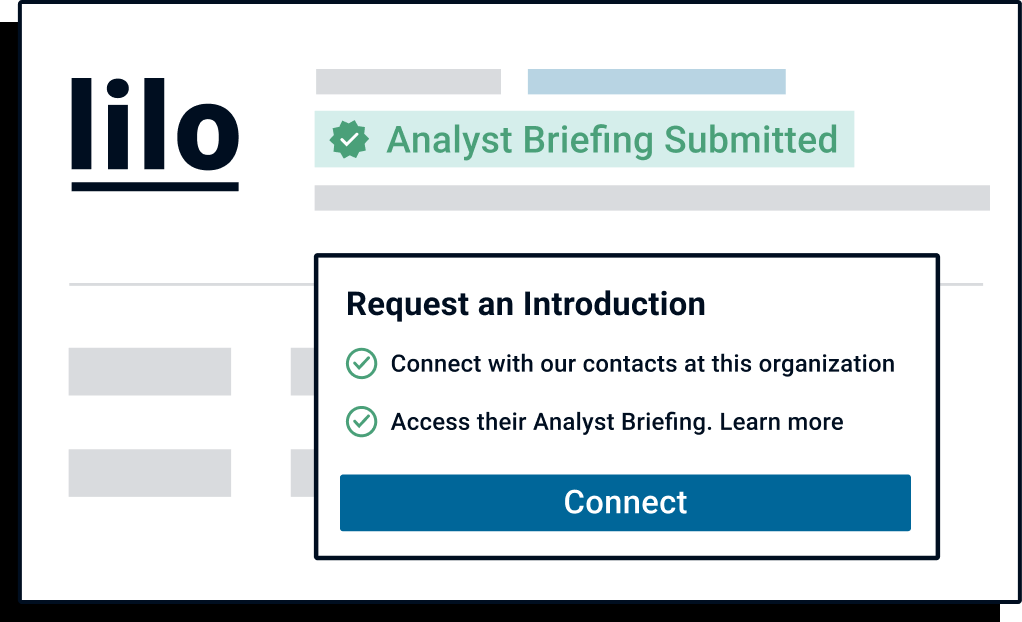
想通知投資者類似麻省理工學院媒體實驗室關於你的公司?
提交您的分析師簡報,以便在CB Insights的平台上麵對投資者、客戶和合作夥伴。188bet游戏
麻省理工學院媒體實驗室的研究
從CB Insights情報部門獲得數據驅動的專家分析。188bet游戏
188bet游戏CB Insights情報分析師提到了麻省理工學院媒體實驗室3 188bet游戏CB Insights研究簡報,最近在2022年3月9日.
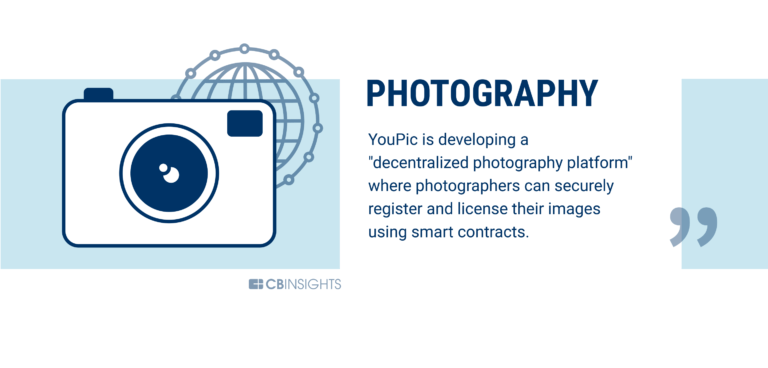
2022年3月9日
銀行業隻是一個開始:65個大行業可能會轉型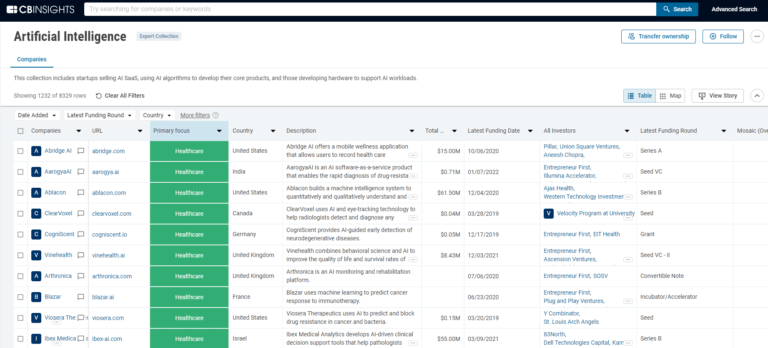
2022年2月21日
5家處於早期階段的醫療AI初創公司,擁有令人印象深刻的創始團隊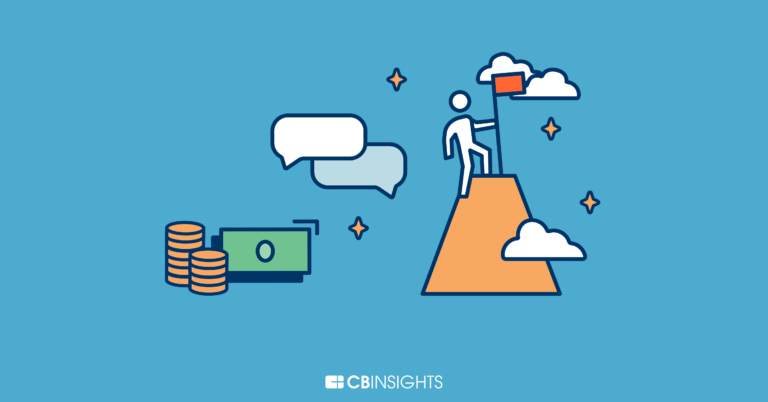
2021年1月21日報告
來自風險投資家弗雷德·威爾遜的14個教訓最新的麻省理工學院媒體實驗室新聞
2023年3月10日
如果我們跳過靜態幀的概念,而是直接分析來自相機的數據流,計算機視覺可能會更快更好。至少,這是麻省理工學院媒體實驗室的最新創意——Ubicept——正在運行的理論。大多數計算機視覺應用程序的工作方式都是一樣的:相機拍攝一張圖像(在視頻的情況下,是一係列快速的圖像)。這些靜止幀被傳遞給計算機,然後計算機進行分析,找出圖像中的內容。聽起來很簡單。但有一個問題:這種模式假設創建靜態框架是一個好主意。對於習慣看照片和視頻的人來說,這似乎是合理的。然而,計算機並不在乎,Ubicept相信它可以讓計算機視覺更好、更可靠,因為它忽略了幀的概念。這家公司本身就是聯合創始人的合作。塞巴斯蒂安·鮑爾(Sebastian Bauer)是該公司的首席執行官,也是威斯康星大學的博士後,他在那裏從事激光雷達係統的研究。 Tristan Swedish is now Ubicept’s CTO. Before that, he was a research assistant and a master’s and Ph.D. student at the MIT Media Lab for eight years. “There are 45 billion cameras in the world, and most of them are creating images and video that aren’t really being looked at by a human,” Bauer explained. “These cameras are mostly for perception, for systems to make decisions based on that perception. Think about autonomous driving, for example, as a system where it is about pedestrian recognition. There are all these studies coming out that show that pedestrian detection works great in bright daylight but particularly badly in low light. Other examples are cameras for industrial sorting, inspection and quality assurance. All these cameras are being used for automated decision-making. In sufficiently lit rooms or in daylight, they work well. But in low light, especially in connection with fast motion, problems come up.” The company’s solution is to bypass the “still frame” as the source of truth for computer vision and instead measure the individual photons that hit an imaging sensor directly. That can be done with a single-photon avalanche diode array (or SPAD array, among friends). This raw stream of data can then be fed into a field-programmable gate array (FPGA, a type of super-specialized processor) and further analyzed by computer vision algorithms. The newly founded company demonstrated its tech at CES in Las Vegas in January, and it has some pretty bold plans for the future of computer vision. “Our vision is to have technology on at least 10% of cameras in the next five years, and in at least 50% of cameras in the next 10 years,” Bauer projected. “When you detect each individual photon with a very high time resolution, you’re doing the best that nature allows you to do. And you see the benefits, like the high-quality videos on our webpage, which are just blowing everything else out of the water.” TechCrunch saw the technology in action at a recent demonstration in Boston and wanted to explore how the tech works and what the implications are for computer vision and AI applications. A new form of seeing Digital cameras generally work by grabbing a single-frame exposure by “counting” the number of photons that hit each of the sensor pixels over a certain period of time. At the end of the time period, all of those photons are multiplied together, and you have a still photograph. If nothing in the image moves, that works great, but the “if nothing moves” thing is a pretty big caveat, especially when it comes to computer vision. It turns out that when you are trying to use cameras to make decisions, everything moves all the time. Of course, with the raw data, the company is still able to combine the stream of photons into frames, which creates beautifully crisp video without motion blur. Perhaps more excitingly, dispensing with the idea of frames means that the Ubicept team was able to take the raw data and analyze it directly. Here’s a sample video of the dramatic difference that can make in practice:
麻省理工學院媒體實驗室投資
4投資
麻省理工學院媒體實驗室4投資.他們最新的投資已經到位SendFriend作為他們的一部分種子VC - II在2019年2月2日.
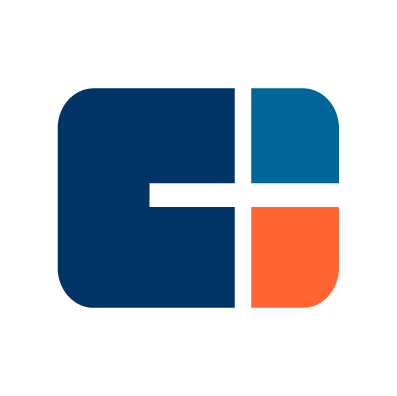
麻省理工學院媒體實驗室投資活動
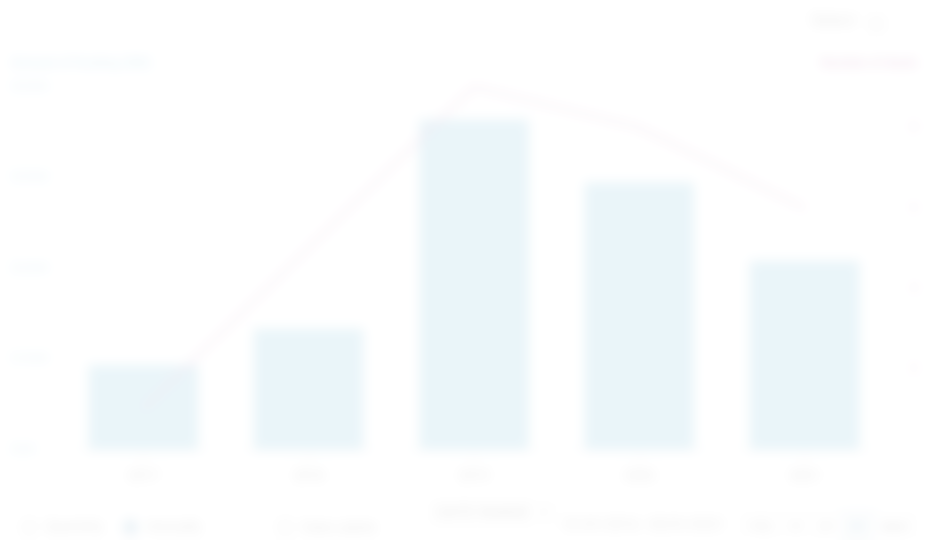
日期 |
輪 |
公司 |
量 |
新的嗎? |
共同投資者 |
來源 |
---|---|---|---|---|---|---|
2/11/2019 |
種子VC - II |
SendFriend |
1.48美元 |
是的 |
3. |
|
11/10/2011 |
兌換的注意 |
|||||
8/4/2011 |
甲組-第二組 |
|||||
7/15/2005 |
私人股本 |
麻省理工學院媒體實驗室投資退出
45個投資組合退出
麻省理工學院媒體實驗室有45投資組合出口.他們最近一次退出投資組合是擎天柱騎 在2022年1月11日.
日期 |
退出 |
公司 |
估值
估值由公司提交,從政府文件或新聞中挖掘,由VentureSource提供,或基於可比較的估值模型。
|
收購者 |
來源 |
---|---|---|---|---|---|
1/11/2022 |
Acq -人才 |
擎天柱騎
|
2 |
||
12/6/2021 |
反向收購 |
Buzzfeed
|
17 |
||
5/25/2021 |
收購了 |
Affectiva
|
16 |
||
日期 |
1/11/2022 |
12/6/2021 |
5/25/2021 |
||
---|---|---|---|---|---|
退出 |
Acq -人才 |
反向收購 |
收購了 |
||
公司 |
擎天柱騎
|
Buzzfeed
|
Affectiva
|
||
估值 |
|||||
收購者 |
|||||
來源 |
2 |
17 |
16 |
麻省理工學院媒體實驗室基金的曆史
1基金曆史
麻省理工學院媒體實驗室有1 基金,包括比特幣開發者基金.
截止日期 |
基金 |
基金類型 |
狀態 |
量 |
來源 |
---|---|---|---|---|---|
3/28/2016 |
比特幣開發者基金 |
|
|
0.9美元 |
2 |
截止日期 |
3/28/2016 |
---|---|
基金 |
比特幣開發者基金 |
基金類型 |
|
狀態 |
|
量 |
0.9美元 |
來源 |
2 |
麻省理工學院媒體實驗室合作夥伴和客戶
合作夥伴和客戶
麻省理工學院媒體實驗室10個戰略合作夥伴和客戶.麻省理工媒體實驗室最近與加拿大銀行在2022年3月3日.
日期 |
類型 |
業務合作夥伴 |
國家 |
新聞片段 |
來源 |
---|---|---|---|---|---|
3/18/2022 |
合作夥伴 |
加拿大 |
2 |
||
1/1/2020 |
合作夥伴 |
瑞典 |
Cardo Health擴展到墨西哥與麻省理工學院媒體實驗室社會影響的副產品- Cardo Health Cardo Health正在與麻省理工學院媒體實驗室的社會影響子公司Prosperia合作,將數字醫療帶到墨西哥。 |
1 |
|
7/16/2018 |
合作夥伴 |
美國 |
這可能是IBM研究團隊在繼續與麻省理工學院媒體實驗室合作的一個更廣泛的項目中探索的問題的一部分,該項目專注於工程道德推理。 |
1 |
|
4/2/2018 |
合作夥伴 |
||||
9/7/2017 |
合作夥伴 |
日期 |
3/18/2022 |
1/1/2020 |
7/16/2018 |
4/2/2018 |
9/7/2017 |
---|---|---|---|---|---|
類型 |
合作夥伴 |
合作夥伴 |
合作夥伴 |
合作夥伴 |
合作夥伴 |
業務合作夥伴 |
|||||
國家 |
加拿大 |
瑞典 |
美國 |
||
新聞片段 |
Cardo Health擴展到墨西哥與麻省理工學院媒體實驗室社會影響的副產品- Cardo Health Cardo Health正在與麻省理工學院媒體實驗室的社會影響子公司Prosperia合作,將數字醫療帶到墨西哥。 |
這可能是IBM研究團隊在繼續與麻省理工學院媒體實驗室合作的一個更廣泛的項目中探索的問題的一部分,該項目專注於工程道德推理。 |
|||
來源 |
2 |
1 |
1 |
為您的團隊發現正確的解決方案
CB I188bet游戏nsights技術市場情報平台分析供應商、產品、合作夥伴和專利的數百萬個數據點,幫助您的團隊找到他們的下一個技術解決方案。